Reimagining Insurance Operations with AI-Native Automation
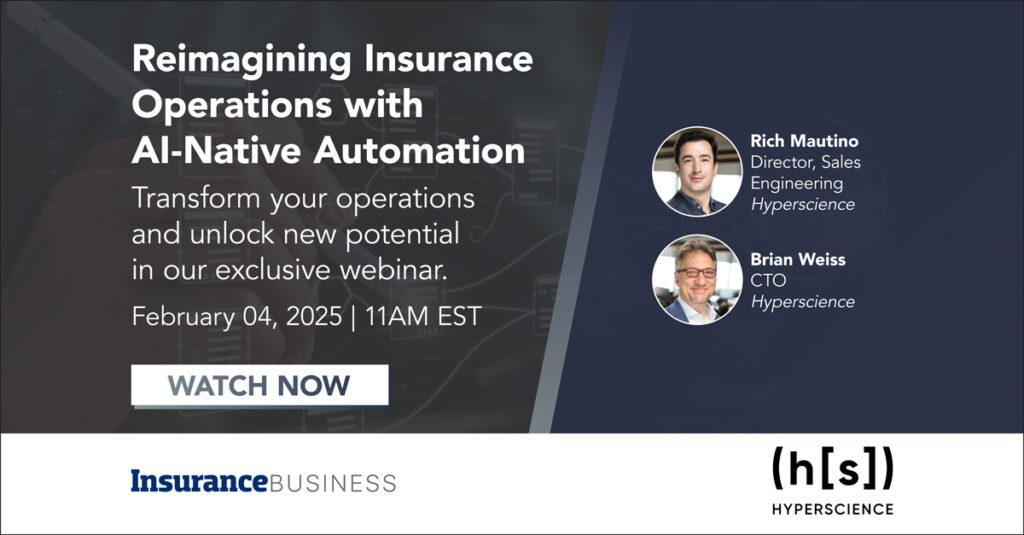
Gia Snape 00:00:00
Hello and welcome everyone. Thank you for joining us today for our webinar reimagining insurance operations with AI native automation in today’s fast paced and rapidly evolving market, insurance companies that rely on outdated legacy systems and sluggish BPO models face increasing challenges to keep up with rising customer expectations. Experts from hyper science are here today to tell us how insurance insurers can unlock new efficiencies and competitive advantages through AI native automation. I’m Gia Snape News Editor at Insurance Business, and I’ll be your host for this session. We are so thrilled to have you with us before we dive in a few housekeeping items, this session will run for an hour with 45 minutes of presentation, followed by 15 minutes for a live Q and A at the end. Feel free to drop your questions in the Q and A box at any time, and we’ll address as many as we can in the dedicated segment. And finally, this session is being recorded, so you’ll receive a link to the recording afterward. So let’s get started in a world where customer expectations are higher than ever, inefficiencies and back office operations aren’t just an inconvenience, they’re a direct threat to stick to staying competitive, legacy systems and manual processes often lead to poor data quality, delays and costly operational models that prevent insurers from making smarter, faster business decisions. But there’s good news. Advances in AI native automation are transforming the insurance industry, and today, we’ll explore how hyper sciences platform addresses these challenges, enabling clean, accurate data extraction that drives ROI streamlines workflows and improves decision making, and to help us reimagine what’s possible in insurance operations, we Have two incredible industry experts with us today. Brian Weiss CTO at Hyperscience. Brian is a long time technologist and has held executive leadership positions throughout his career. He has extensive experience developing deep expertise in structured and unstructured data, creating roadmaps for AI and machine learning product offerings. We also have Rich Mautino, Director Sales Engineering at Hyperscience. Rich leads the pre sales organization for North America and has worked across numerous industries and verticals, from Fortune 100 companies to fast growing technology startups. His team helps customers achieve real world business outcomes across dozens of verticals and use cases. Gentlemen, thank you for being here today.
Brian Weiss 00:02:49
Thanks, Gia. We’re glad to be here. Hi everybody. I’m Brian, and I’m joined by Rich Mautino over there in the black background, we always have to flip a coin about who gets the light side of the force and the Dark Side of the Force rich does look more and more like James Bond every time we do one of these. So you know, if you’re tracking his his profile photo. But look what we want to do today is we’re going to we’re going to talk about some key trends that are impacting insurance operations, and sort of where we see those going in 2025 and that’s comes from perspective of how we invest in technology and what our current customers are doing. We’re talking a little about our approach to an AI led modernization of insurance processes. Rich is going to do a demo, and then we’re going to get into some recommendations that you know, from the seat of hyper science, where we see and where we would recommend folks to lean in before we get into a QA but before we do that, let me just let’s talk a little bit about what hyperscience is. If you don’t have any background on the company, we are roughly a 10 year old company, and we are a pioneer in AI and ML led automation. The company was founded by data scientists, by data scientists and ml engineers, roughly 10 years ago, who having sold their first company to Sound Cloud, turned their attention to the hard problem of human information inside the enterprise. How can you get a machine to understand like a human, what it’s looking at and efficiently and accurately move that through a business process, effectively creating a digital worker inside the enterprise. What they realized was that if first of all, the only way to solve that problem is through machine learning, right, you can’t apply a rule or set of rules to try and understand something as complicated as handwriting. And they did this long before. I mean, Bert didn’t exist for all you tech folks out there, and certainly way before any transformer papers. But the idea that you could use deep learning to bring a human like understanding to the problems in the back office was the founding principle of hyper science. We’re a product led company. We spend well over half of our R and D budget, our budgets on R and D, and that’s primarily a. Around data science and machine learning expertise. We’re very well funded. We raised roughly $300 million over that period from tier one investors stripes Tiger and Bessemer being the top three. We have an array of blue chip customers who have been with us for many years, many of them at this point processing. You know, for example, the VA does a billion documents a year through hyper science systems. So we are, we are quietly embedded in some of the largest processing operations that are happening out there in the market. We’re also tied to a partner ecosystem, folks of like IBM, Accenture, Deloitte as well as partnered with AWS, Microsoft, Google as well, needs to be updated and and so that’s kind of a framework where we are. We are really pioneering the concept of hyper automation with AI by building sovereign models with customer data. So what, what you’ll hear about is the platform we bring to the market, which allows our customers to use their own data along with hyper science to build high performant models for automation, 99% accuracy and 98% automation, right? That’s a huge shift from transitional technologies or traditional technologies that have just kind of used blunt force to get it done. Now we also play in the insurance industry pretty significantly rich go the next slide for me, strong track record of the insurance industry. Some of our customers are listed here, and it’s not. It’s not hard to imagine why that’s the case, right? Things like underwriting, claims, processing, enrollment, those that those bread and butter functions of the insurance industry, how a lot of human information embedded in them and a high amount of variability that doesn’t ever it’s very hard. I mean, we’ve been trying to systematize the chord forms, and that’s but there’s still so much variation. Never mind, even before you get into having to deal with handwriting and complexities of multi language. But those use cases for us end up being spots where companies like the ones listed on the left, you know, they move their automation rates from from 70% an accuracy of 60% with lots of BPO spend and long tails of efficiency, of inefficiencies, to 99% right? So really, much faster. And then there’s some of these use cases that are cracking open even more for us now, claims management, fraud detection, which are really about long form. You’re not just looking at a key value pair, an orchestrated, you know, system, but you’re looking at understanding what’s in a 5060, page document narrative, maybe looking at understanding the patterns that might indicate fraud. So these are use cases that we hyper science delivers into the market for our insurance customers. Rich. I think we want to do look, let’s kick it off right out of the gate here, because we would hate for this not to be interactive. What you know for for those of you on the line, real quick, top of Top of Mind here, if you had to classify where you’re experiencing the most trouble in your operations. What would you call it? Is it still enrollment to underwriting claims, or are we getting into contract management, fraud detection and overall claims management? Quick, quick answers. I don’t know. Rich, you got to vote here. Come on, man. What do you think? What do you think they’re going to say?
Rich Mautino 00:08:18
Yeah, and I know they are. They’re all wondering, you know, is there an all of the above button? And yes, there is. That’s why we’re here. But I see a lot of claims processing and claims management out there. A lot of our customers have explained that, you know, there’s a lot of systems out there where information flows in and out of these systems and seamlessly allowing the data and the information the decisions to be consistent across those is a challenge underwriting,
Brian Weiss 00:08:43
Yeah, lot of crunchy data and underwriting, absolutely, that’s a good one. Thank you all for jumping in and engaging here. So next Rich, I think what we want to do is talk a little bit about the 2025 what we see is the insurance trends and priorities specific to AI automation and those sort of things?
Rich Mautino 00:09:01
Yeah, absolutely. And we know that these processes rely on billions of documents every year, and there’s oftentimes a lot of different kind of goals and functions there, ranging from onboarding to auditing underwriting, which nearly half of you said was a big one, all the way to fraud investigation, claims processing and then renewal and endorsement, and all of the content you see at the bottom is kind of what feeds up into that. Some of these go into all of them. Some of them are one to many, many to one. And the end result is this, which is that half of the business processes out there today are still paper based, and that’s from deep analysis. And we see that out in the field. We probably see even a little bit higher than that, but ultimately, 90% of the data that our customers show us is unstructured and not machine readable, so it presents great challenges to taking it from current state to future state, and that’s where we get really excited about problems that we can solve.
Brian Weiss 00:09:56
Well, yeah, and look, if you ask analysts who go and ask both CI. CEOs and CTOs and business leaders what they’re going to invest in in the insurance industry, there’s some not surprising, not unsurprising answers here that 68% of CIOs are planning on focusing on modernization. Yeah, go legacy systems. Of the opportunity to modernize is there, if you ask somebody like Forrester, for example, what you know, what decision makers will apply AI to its automation and efficiencies, right? So people are beginning to understand the possibility that AI offers for automation. Now, the last stat really is most interesting, and if you look at this study from Accenture, that there’s $170 billion of premiums at risk from from customers who switch because of bad experiences, right? This question of how satisfied were, were you with the company who handled the claim? If you take more than six months or let or three to six months to get that work done, your risk of losing that customer? So there’s this combination of needing to be able to cut costs, yes, be more efficient, yes, but the key factor is, what’s happening at the top line, am I? Am I driving customer adoption? Am I creating a better experience? And look, creating a customer experience means faster claims processing. So it’s not just about, Hey, did you get better accuracy and did you get things right? But how fast can I move that experience through, right? So where are my manual processes? What do you got to look for? How do I automate workflows that are stuck, like every single place where somebody has to touch it? That adds why? In order to do that, you need to create operational efficiencies, right? So automating workflows requires what Rich operational efficiencies? Let’s look at what’s inefficient and why? Right? Where does automation create errors? Errors are incredibly important to look at, because once they get further downstream, they get compounded in the impact of how to solve them, right? It’s why something takes six months. Is because something went wrong in the process. Right? The criticality here is high data accuracy, right? I hate to say, garbage in, garbage out too often, but really, like you need to think about what is getting, how you were getting data, and the accuracy of the information that drives it. Now, the systems that drive accuracy of information are the problem. We are right now at a transition point in technology, really, where the opportunity to shift from rules based approaches, which would try and throw a template and don’t match, and then throw dump everything out to a BPO if it doesn’t like they can’t do the kinds of work that people do so automating to a model based approach. First one that you can actually train yourself where you have visibility of the accuracy is where you are going to unlock Gen AI or potential. Whether it’s Gen AI or others like using AI to do what people do in a controlled manner to drive high accuracy is what gives you compelling customer experiences.
Rich Mautino 00:12:50
Time for another poll. We’d love your input, and this time, we’d like to know what’s been your main approach to improving the customer experience, or what is that plan for 2025 you know, is it optimizing allocation, you know, trying to be smarter about what resources you do have. Is it replacing legacy applications, kind of ripping out the root of the problem in some cases? Is it automating manual processes, trying to connect the dots and get things you know, working smarter, not harder? You know, ultimately reducing claims process time is rooted in a lot of this, but that focus can be a big one for a lot of our customers, or is it to create that AI copilot experience? A lot of our customers out there, Brian, you’ve probably seen it as a CTO out in there, in the field, are trying to create that co pilot experience and kind of do the best of both worlds. Yeah.
Brian Weiss 00:13:36
How do you drive a genetic AI type thing? Well, yeah, again, we didn’t put an all above for a good reason. Here. We’d love to just kind of get a nuanced answer from you all. I think all the above is a great answer, but I’m interested in how people think about the balance between cost savings and customer experience, right? So what you’re what are you eventually driving like, what’s the top down approach? Yep, okay, 70% for automation. Drill site. Man, let’s go figure out where people are working that we could possibly automate that. All right. So what we want to talk about is how hyper sciences approach to AI and ML. And I mentioned that we are born as an ML company. So what I want to use to frame it up is basically an old versus new, if you think about it. What we are trying to do is take all of the complexity that’s in things like the documents to the left, and that’s not just in structure. It’s also in content. What’s what’s handwriting, multiple languages on stamps all over it. People spill coffee on it. Like, how do you, how do you get the variability of these chunked down into a place where you can then push it through to an insurance workflow and make some decisions in the fastest way possible. The origin in this industry of the technology is document centric. Now, what that means is that you’re taking anything on the left and you’re trying to match it, what Rich to a template or some sort of a you know? And what happens is, is you end up creating these exception queues because of that, because the systems that do. That work, don’t really do it very well, right? So if you’re matching, you know, you’re trying to match a template, you will never chase the variability of the world by matching yet another piece of variant here, right? You end up with your passfail exceptions, and then you can’t get that. And then, of course, downstream, your SMEs have to catch the exceptions. The amazing thing about the legacy way approach to this is you, you have this black box that thinks it’s right, right? And it’ll, it’ll punch out 90 out of 100 documents that process, but, and it’ll say it’s STP for 90. But if you read the documents that came out, they’re wrong, like there’s no accountability for accuracy in those systems. They’re just kind of blunt, right? And so what you end up with is, is this assumed accuracy, which, course, isn’t there. And then you need to dump the whole thing out to a BPO, after you’ve built your own accuracy harness to try and figure out if it got it right or wrong. And so this, this blend between, you know, find the cheapest people possible to clean up the mess and try and make a black box technology get better over time, is kind of where we’ve been stuck for a long time, right? And I think that really caps out at 60, 70% accuracy in automation, sometimes even the more complicated the documents, even less than that, very expensive and above all, slow, so rich. There’s a new way to think about this, which is you take machine learning at the core of it, and you train models plural to do what people do when they look at a document and say, Hey, what is this? Here’s a box of documents. Classify them for me, by the way, take the ones that you’ve got, and I need you to find these 15 pieces of data. But they might live anywhere, and I’m not going to tell you where they live, and I’m not going to give you an x y coordinate. Just go find them. And once you’ve found them, what I want you to do is is actually read the handwriting and tell me what’s in there and get it right. Now. What happens with that is that is that you, what hyperscience is doing is giving customers a platform to build and train these digital workers on their own data. Right? You start with our technology, and you build up these models, which become incredibly accurate, not only they accurate, but they’re also accuracy controlled, right? So you have the ability to make them better. And that’s a critical question. When you’re talking about AI, somebody says, Hey, I’m going to give you a model. Your first question should be great. How do I make it better when it’s wrong? And if they can’t answer that question, move on. I mean, there are lots of tools out there. A model is a tool. The question, do you have a system that allows you to make it better with your data? Can you see when it’s wrong, why it’s wrong, and improve that process? Now, the other thing that we’ve done at hyper science is change the approach to humans and machines. Right? We’ve taken a just in time human loop approach to accuracy. So instead of the black box dumping it out to you and you figuring out if it’s right and when it’s wrong, you pay a BPO to do that work, the model, think of it as digital worker will actually raise its hand when it can’t meet your accuracy standard. I’m a little bit confused between the A and the u here, and I’ve analyzed the sentence and it makes sense in both cases. And you’ve told me that I can’t, I can’t get below 99% accuracy on this. I’m going to ask a human for help on this one spot, right. And by UN clicking that you take it, you use a fraction of the labor that you would use on a spend on a BPO to do the whole document, to sit next to the machine and unstick it in that very specific place, so that changing the mix. So you’re thinking about how humans and machines interact to get an outcome like 99% is really what hyper science is driving. We have been pioneering that approach since the origins of the company. It makes a huge difference. So those are some key things to think about as you look at into AI for automation. It’s about an ensemble of tools. It’s about controlling accuracy. It’s about having visibility on a on a on a platform that allows you to constantly improve right? Always ask the question of, what does that model do when it’s wrong, right? What do? What do you do with with wrong out the other end of it? So Rich. Next couple of examples, right?
Rich Mautino 00:19:08
Yeah. Ultimately, all of this is exciting, but if there’s not results, it doesn’t mean anything, right here, what is the so? What of it? And we’ve got a few very diverse examples that we wanted to share of real world outcomes that we’ve achieved. So with legal in general, general, we started with them five plus years ago to streamline their automation in the insurance workflows. So 70% of you said that automating manual processes was a priority. That’s what legal in general said as well. Back in 2019 when we came on board there their their customer experience, they say, has never been better, and as a result, they’ve won the money facts consumer award the last five years in a row as the life insurance provider of the year. So we like to think that it’s no coincidence that that award coincides with their streamlining of these processes with hyperscience Another in a difference. Scenarios in the public sector. The VA is one I can relate to personally as a pilot in the military. When I did my first VA claim years ago, it took nearly two years for me to get my initial application to the time I actually got even an appointment to be evaluated. So with 9 million plus veterans, you know, processing billions of pages. You can imagine the scale and the importance of this. We’ve got the VA Secretary quoted as saying that processing has never been faster. You know, those claims are the fastest in history. And I can agree with that from firsthand experience, because just last year, they periodically do reassessments and they look at everything again. And I almost thought something was wrong, because from the time it started to the time it ended was less than two weeks, and coming from years to two weeks, I thought something was wrong. I thought, yeah,
Brian Weiss 00:20:52
oh, somebody must can’t. Cannot have happened that fast. I must have somebody made a mistake in my favor. Yeah, it’s exactly pretty astonishing results we’ve gotten there.
Rich Mautino 00:21:00
And then finally, with core bridge financial they retired a lot of legacy tooling as well, and what that resulted in was a 70% reduction in data entry time that took them up to 95% accuracy in handwritten forms, and that’s coming up from 10% so really putting context, it’s over 1,000% ROI when
Brian Weiss 00:21:19
they were not a typo, right? Everybody looks at that. Wait a minute, 101, somebody put an egg. No, it’s not, it’s a well over. I mean, the way they have paid, you know, for hyper science, many, many, many times over, with efficiencies. And that’s actually not uncommon, the kinds of kinds of ROI we see
Rich Mautino 00:21:35
Exactly. So, you know, one of their executives was quoted as saying, it completely transformed the way they do things, and it’s never been easier or more efficient, with 1,000% plus efficiency. You can see why.
Brian Weiss 00:21:48
The thing about Corbridge, I like, is, you know, they actually transition to a different approach to people and machines, that bringing that human in the loop and just putting a fraction of that labor next to the machine, as opposed to the traditional model of, you know, BP over here, and and and black boxes on the other side. Look, we’re talking about trends. And so I want to, I want to comment about some recent, you know, work by Gartner here, very much aligns with with hyper sciences strategy and where we’ve been investing over the last 10 years. First of all, no secret, Gartner will tell you, models plural are the future, right? So it’s not really about there’s going to be one magic model that you can plug into that’ll fix it. The future is about a portfolio of models like to get work done. You don’t need a billion parameter model to get the square root of 64 I can get you a very, very efficient answer with a small language model that’s built on your data, and then I could go ask that model really tricky questions, like, what’s this customer’s intent? Right? Are they mad? Are they not? Like, what’s going on? So there’s the this concept of using AI in an ensemble portfolio approach that which is absolutely the future, right? Because otherwise you could be using a gold plated chainsaw to, you know, to hammer a nail, cost wise, and otherwise, what that means is model ops now becomes very important. So, you know, models are tools, a platform that allows you to orchestrate these tools in a way with visibility, transparency and, and, and, you know, continuous improvement is where the market is moving. I think it’s pretty obvious that rules and temples don’t work. I mean, they work for a while, and then the temple changes. You got to pay somebody to change it. So you need to move through that old legacy technology. And they said, really, you know, their their their comments about hyper science are really accurate. Like, we have deep, deep expertise in machine learning. We ship well over 30 proprietary models out of the box, allowing you then to fine tune those with your own data and also integrate to third party models like llms in a safe and secure way, because we wrap the same kind of accuracy controls around those third party models that you that we provide with our own models that customers Build just other, other, you know, we are now recognized across the board by most analysts as leaders. Gartner has yet to put out a wave, but the Forrester, for example, is one I’m particularly proud of, because it’s about the core technology that’s under the hood, right? This is document mining and analytics. And you’ll notice, we’re just a hair, a hair’s breadth to the left of a company called Google on strategy as far as market leading, and this is from a field of hundreds and hundreds of vendors to get cut down to this top four or five. IDC as well, sees us Giga ohm. And then, even just from a feature function perspective, folks like ISG, who, you know, ask people to rank feature functions put us well on the lead, even above companies like ServiceNow for document processing, in particular, again, all of our technology. It’s our technology embedded. We’re not OEMing anybody else who’s on the wave here as part of the core tech.
Rich Mautino 00:24:51
So I know everyone’s probably excited to see this in action, kind of see where the rubber meets the road before we jump into that. Wanted to prime that by getting an understanding of what. Approach our customers and our prospects are taking to process documents today, and we did give you a combination of the above answer choice here, yeah,
Brian Weiss 00:25:11
I want to ask you guys, don’t click the last one. Don’t say a combination above could. Course, that’s true, right? But try like, on balance, like, what? Like, what do you where do you spend? Where what’s doing more of the work machines or people? It’s commented about. It’s kind of too easy to say that, right? Rich, maybe we should have adjusted that one next time. I mean, this looked this balance between, you know, paying for people to do work and trying to get better machines is one of those things that, you know, we’re having lots and lots of conversations about completely rethinking that process, right? Yeah, and I’m often involved in the ones where you really need to get up and above the budget centers here, because what I find sometimes is like, the folks who are in charge of buying the technology don’t have any budget for people to put next to the machine. And then there are different budgets for the people who so a lot of times you have to just rethink, you know, reality here in house people, yeah, yeah. Now that would correspond to what we saw. And then from, you know, back at the beginning, about, you know, how much paper and how much variability is out there. Thank you for not all choosing combination above. We appreciate that.
Rich Mautino 00:26:19
Absolutely. So now, without further ado, we’ll hop into a demo, and what I’ll do is, before I jump into this, I’ll kind of set the stage of what we’re looking at here. This is all in the browser, so you don’t have to be a data scientist to use this. This is meant to be something that can enrich your orchestration of processes. So imagine an insurance claim process where all of these documents come in. Let’s say a claimant emails them all in. They go into a system, and historically, they get scattered to the wind. You’ve got people kind of working on them all over the place. Here, what I’ll do is I’ll simulate that coming in here, and when that submission comes in, it’s going to hit the system, and you’ll see it actually start running automatically. So in this case here, you can see the submission just hit. And if I look at the activity log, it’s already off to the races here. So it’s got seven pages in here. It’s an activity log. And what I like to really emphasize here is watching the flow run our blocks. And flow architecture is what I think sets apart. And what you’re seeing here is the true orchestration. So historically, all of these things are happening, but they’re happening in different places. They’re not interconnected in any way. So not only is the accuracy and automation affected, but oftentimes the actual customer experience is getting bounced all over the place. We can all relate to times where, you know, we’ve sent something one place and then get asked for it from a different place. You can see what hyper science is doing is automating a lot of this here. So these blocks I’m going to quickly scroll through and show you all of the things that in the course of this demo are going to happen very quickly here, yeah, things that we can orchestrate.
Brian Weiss 00:27:58
Yeah, and that’s not true, right? That orchestration allows us to do like, dig into the documents. We’re going to call an LM and ask it to summarize, right? You’re going to make a decision. I’m trying to steal in your thunder here. Rich a little bit, but people oftentimes, when I talk about hyperlink, oh, yeah, you do extraction. No, no, we’re orchestrating decisions right in line, and also validations against external systems, right? Data, all of that gets pulled into hyper science now
Rich Mautino 00:28:21
Absolutely and now you can see we’ve got a task ready. This is a human in the loop function that I’ve actually intentionally created. What the neat thing about this is, I’ve got something to show you here, but in reality, what I’m showing you in the real world, we wouldn’t see this happening over and over, because the first time we correct it and we start setting it on the right. Course, we’re not going to keep dealing with that over and over. But in this case here, you can see it’s identified two documents here. It’s still got some unmatched pages here, and it’s raised its hand and it said, Hey, I need some help with these nested tables here. So rather than having you do it from scratch, it’s going to show you what it thinks and give you an opportunity to either say, Yes, I agree, or no, let me help you out here. So in this case here, you can see this complicated nested table. It’s actually done a really nice job with it’s got everything correctly coded. So we can say, Yep, good job. You got that one right. And you can see it will put yellow borders around where it thing it might be wrong. So in this case here, we’re in good shape, but we look at this other clinic here, and we look at this invoice, and we see, actually, it’s, it’s skipped a little,
Brian Weiss 00:29:34
Losing rich. That’s okay. I’m gonna try and narrow it until he comes back on, uh, assuming I’m not the one who’s frozen.
Brian Weiss 00:29:49
Okay, it’s rich who’s frozen, all right? So what you’re seeing here is that the machine is rage here,
Rich Mautino 00:29:53
and it’s kind of, it’s kind of confusing. Then there we go. Are you still there?
Brian Weiss 00:29:59
Yep, we. Got you rich. You’re breaking up a little bit, but I’m narrating, when you break up, you’re good. Yeah, perfect.
Rich Mautino 00:30:04
So you can see it’s recategorized that. And in this case here, I’m going to help it out. So I’m going to draw this first row down to here, and then I’m going to add another row. And this is where I got stuck last time. So we’ll help it out here, and extend it down to this bottom row here, drop it down, and you can see now it’s going to catch everything. We’ll add another one. In this case here, we’ll set that correctly. We’ll add another row. We’ll draw these charge categories here. So you can see, I’ve taken about 30 seconds or so to help the machine along, and now we’re back off to the races here. And so this submission is now running once again. And if I look the flow run, I can watch that happen.
Brian Weiss 00:30:49
Yeah, for was a little bit choppy there, but part of what you guys were seeing right is the ability of the machine to raise when it’s when it’s slightly confused and can’t meet an accuracy standard, and then at the same time, enable you to, very quickly, with the least amount of effort possible, unstick it, and at the same time that, of course, then goes, can go into a training set to make it better. I can’t. I think Rich is frozen again, but looks like he’s working. Wi Fi, you can do it, man. All right, so I’m going to tell you what Rich will show you as soon as this on sticks the flow run that’s underneath this is the various pieces. Ritz, tell me when you’re back. Man, are the various pieces.
Rich Mautino 00:31:26
of that. Sorry, I’ve switched over to it.
Brian Weiss 00:31:29
That’s okay. It’s no fun unless something goes crazy, right? So what, yeah, what you’re seeing then inside a flow run is, is the various steps that are happening. So you saw, it’s trying to transcribe. It’s trying to identify. It’s trying to transcribe. Each of those in hyper sciences, separate model right, rich and and those models then become trained with with customer data to become more and more accurate. And they each have an accuracy threshold that is set such that if you know you can’t meet a standard, it’ll ask for help at that point. But you can see, as you train these things over time, they become extremely accurate. And so I don’t know, rich, where you want to pick up, man, I think you got your Internet back.
Rich Mautino 00:32:10
Yeah, absolutely apologies for that. So you can see here that while that was running, actually, this is the beauty of cloud model here is that while my voice was interrupted, everything is in the cloud. So this workload has actually been working behind the scenes for me. So we’re actually already to our next task, which is this is asking for some help with transcription. So in this case, here you see it is flagged to post code, and it said, Hey, I need some help on this. I’m not positive. And you can see we’ve given it some intentionally, some very tricky information here, is this a five? Is it an S? Is that a one? Is it an I? And what I’ve done for the purpose of the demo is actually trigger this, and in this case, they’re going to correct that that’s a zero. But the neat thing here is that we wouldn’t actually hit this in the real world, because we could either set a data type here, where you can see, I can actually tell hyper science what to expect. I can say, hey, it’s always going to be letter, letter number, for example. So that’s one option. The other is, of course, an API where we can have it connect and actually look and see, is this a valid postal code to begin with? And it knows the answer every single time here. But if I did that, I wouldn’t have anything fun to show here. So I’ve corrected it. You can see in this date, they’ve kind of squished their two a little bit and put an apostrophe. It’s outside of the bounding box. So again, it got a little hung up here, but let’s help it out here. So 24th may 2023 look at all the other stuff, though, that I didn’t need to help it with. So you can see, there’s a pen laying across the screen. There’s a scratch out. This is on a second line. This is all things that it got correct. And it just asked, Hey, I need help with this. One thing. You don’t have to start all over here. So we’ll go ahead and submit that and let that run. And when we’re doing that, I’ll come back to the flow run here. And again, the exciting thing about this is truly being able to see and watch how quickly this goes through a lot of different things. So you can see the manual transcriptions done. Now, what it’s done is loading this admission, starting to build a shell. It’ll grab full page transcript, transcription, and then you can see there’s a lot of other orchestration layers that it’s going to move very quickly through, looking for entity recognitions. You know, is this clinic that we have on file, where’s the policy number. It will collate things. It’ll check for validity of policy. And then finally, we’ve got a fork in the road. Here is additional human validation required. So depending on what your needs are, you can set it up to either say, Hey, I have everything I need to make a decision on my own, and it can actually reference things like a database, look at past claim history and make decisions based off of previous input. Or it can actually raise my hand and say, Hey, I need help. I need some guidance, because making a wrong decision in the insurance world is is very catastrophic for a number of reasons, creates a bad experience. It can be very costly. So this is replaced. Seen the historical process where, you know, an analyst would come to the boss and say, Hey, boss, here’s the packet. I’ve got everything all kind of highlighted and noted out. Here’s what I found, here’s the background. They would explain it all, and they would look through it and make a decision. We’ve automated that experience here. And you can see what the supervisor got. The has got the ability to do is quickly review all key information. And they’ve got that sort of AI copilot experience here on the right. So this is what we call custom supervision. So in this case here, if I need to make a decision, rather than having to look for everything, it’s all where I need it. So in this case here, I got my policy number. Looks like the policy started back at the end of March of 2023 here’s the patient name. By the way, it looks like they’re a little unhappy. We’ve got some negative sentiment here. So behind the scenes, we’ve kind of gathered that from the email, and what it’s doing is making a recommendation and telling us why. So in this case here, it’s saying, hey, this claim should be rejected. And the reason why is that the first symptom of this actually occurred before the policy start date. So essentially, you can’t go get a policy and then make a claim for something that was, you know, in existence before that. And in this case, it’s saying, here’s the first symptom. So I can click on that, and it will actually bring me right to where it found that. That way, if there’s a mismatch here, I can say, Oh, actually, no, this is actually good to go in this case, though, you can see that the first symptom occurred back in February, and they started the policy a month later. But furthermore, there might be pause policy clauses that come into play. Maybe there’s a grace period or window here. In this case, though, hypersite has gone into their policy, here, and then furthermore, it’s taken a very lengthy doctor’s note, which sometimes would need to kind of be looked through. You know, you can read through the emails the doctor’s note here. In this case here, it would just summarize that for me. So I have everything I need here to make a decision. And what I can do here is, click, reject the claim. I can put the notes in here, and I can put case notes, you know, pre existing policy symptom. So in this case here, now this can go in a vector database, and in the future, if there’s similar claims made to this, we can expedite the decision and also get to that more accurate. So in this case here, it’s going to continue along its way. What we’ve got in place is actually redacting the documentation as well downstream. And this is what our end result here, which is 100% accuracy on the field identification, 98% on the table. And that was a very complicated, nested table, as we saw, 98% of the transcription. And that’s actually a little unfair, because I tripped it up intentionally, so we would be pushing probably closer to 100% there. And ultimately, you can see all of this was orchestrated and sent downstream in one singles experience.
Brian Weiss 00:37:57
Yeah. and the cool thing, right? I mean, you have visibility into where your accuracy is, and you have visibility into where it’s tripping up. It’s even down to the field level, right? So you can say, I want this field to be more, you know, I care more about accuracy on this one, then maybe the check box for, you know, please don’t send me advertising. Always get it wrong. They send me advertising anyway. So your ability to get very, very deep into these documents and to see where, like you can these models get trained to be very, very accurate with a light touch by by not data science users, these are business users who can, who can fine tune and refine the performance of the models, if and you’ll notice, what Rich also did was he combined it with, Hey. I mean, I need to go summarize some data, right? Or I want to go read this policy and pull out the key, you know, narrative information from we’re coupling with LLM systems, right? So the ability to use an ensemble to get a chunk of work done in the most efficient way is part of what hyper science delivers. All right? Just some quick technical advantages, because I’m from CTOs perspective. I can’t, I can’t, not talk about it. We were born as an AI company. We’re born ml. We would not start as a, you know, a legacy OCR tool that just figured out how to start using ml. That’s the whole premise of the company. Is forward. The concept of orchestrating accuracy is novel to what hyperscience does? We do it in a different and embedded way. We don’t just give you a confidence score and, hey, go figure it out yourself. We actually orchestrate a complete accuracy of the end product and bring you into the loop when it’s necessary to that particular field, so you don’t go have to figure out how to fix it later. A big part of our success is that it’s built on a turnkey platform. So this entire platform for model operations, for orchestrating models, for orchestrating accuracy for reshaping how human humans interact with with machines, around documents. That’s a single platform to take it really beyond your IDP process we orchestrate bringing other data in. In that example, rich just gave you like we reached out to various sources to understand where this claim came from, etc. Brought that all, all of that data to. To the point where you’re sitting with the documents right to make a decision. So you’re utilizing AI where it where it has the highest impact, right in unsticking these processes. By the way, it’s delivered turnkey, but we deliver both on prem. You can buy software and run it. We have, you know, highly regulated businesses who do that. We also run a private cloud or SaaS business. As of last month, we are FedRAMP High certified. So il five data included. So we operate in really rarefied, secure environments with data. Rick, one couple more clicks here. Rich, you know, people sort of sometimes ask, well, What model do you use? Yeah, we use all of them. When there are 40 models embedded inside hyper science that you never see, including handwriting, all of the work that goes on before any any of those blocks happened, we split out things like classification, identifying and transcribing into tunable models. And then we can plug in models that, that that, that you know, that help that process along in the most cost effective way, right? So, but our extraction is absolutely world class, like our handwriting is. Our work on transcription is the best in the industry, and continues to stay that way. And then, and then, for last, also rich. One thing you pointed out here that I’ll just raise a differentiator for on this next slide is that is that enabling orchestration to help AI make for decision support like it’s one thing, hey, I’m gonna go use a model to get an answer. But can I embed that model into an orchestration process that also uses models? Right? Because, don’t you forget that if we’ve got a human in the loop, you better believe we’ve got models. Got models in the loop, right as the next phase. And then how do we get that to work? So you know, you gave us some great examples, rich of that in the in the demo here, but talk about this a little bit more before we move on.
Rich Mautino 00:41:52
Yeah, we see this all the time. And the neat thing is that this is truly customizable, so we have customers that get inputs that are in foreign languages, for example. So having an AI based decision, not only be able to present it where it’s translated, but then summarizing it, for example, allows people to make better decisions faster. So we’re taking a huge step further from the traditional just classify, identify and extract, doing a lot of really valuable stuff behind the scenes that allows you to, you know, kind of let the AI do the the time consuming, monotonous stuff, so that the human can make a better decision with better inputs.
Brian Weiss 00:42:30
Yeah. And it’s a full orchestration platform. I keep coming back to that because I see a lot of, oh, we’re going to buy this model and hire some people to do some things that, like, okay, let’s think about the whole end to end process, and how you build in model operations into your forward thinking about AI for your for your document processes. A couple more slides here, and then we’ll go to we’re going to just close it out for some questions. If I haven’t already mentioned it rich on the next slide, we, you know, we operate at the highest enterprise standards, not just for for throughput. I mean, a billion documents at the VA is not trivial. But you know the types of security accreditations we have. I mentioned FedRAMP, high 24/7 worldwide technical support. So really, and then we, we take our sort of the AI and the ethical pledges around AI very seriously, part of the core of our DNA is bringing ML and AI to the back office in a secure and manageable way. Like, where is your data? How is it being used? And then, so if let’s take the last slide here, Rich, I know that we’ve got some sort of just, you know, some thoughts, predictions, suggestions, and I will pivot off of that, like, how do you use AI in the insurance industry, you know, to its best impact right now, right? And I think it’s important, if you think about some of the, some of the things we’ve opinions we showed you from Gartner and others like, you have to cut through the hype a little bit here, right? I think we’re now into this maybe, maybe the one and a half or second inning of a very long game, the first one being, everybody’s excited about, oh, the frontier models are going to do everything, and it’s going to be magic, and they didn’t know exactly what people do. But you don’t generalize. Frontier model is the wrong tool for the job in many cases. And again, it’s just a tool. It doesn’t tell you when it’s wrong. It doesn’t tell you when it’s confused. It doesn’t tell you what to do with the outputs. It doesn’t orchestrate anything after that. So a lot, what we hear a lot right now is AI is really a solution looking for a problem. We understand desperately that there is an opportunity in the future for an AI, say agent, to understand everything it needs to know about my business, and be able to answer my claims adjusters questions and all of that. But it’s predicated on one thing I need to feed it the language of my business. I mean, I think the other day it was lots, lots of us think about this, but some of these frontier models, there is no more public data to train them on. It is gone. The gold mine for AI is inside the enterprise. The problem is. It’s private data, right? And so hyper science has been able in customers to get to that and leverage that information for 10 years plus in service. I would say a couple of things. Look for very practical applications. And if you haven’t figured it out by now, document automation is a, is a, is a layup drill site for AI and do it properly. Don’t just throw it at a model and say, Look what I got and then try and hire a bunch of people to write prompts to see if they can get it right or wrong. Like, that’s a that’s a whole different problem, but, but how look at where your people are doing work that you don’t think they need to most of you said that you were, you know, you’re doing this in house with people. Okay, is it on simple or on complex documents? Okay, don’t, let’s not be afraid of the complex documents, but look at where you’re spending money on where is the friction? Where do you spend money on a BPO? Where do you spend money? What slows your process down? And start there it is. Hyper science is one of those applications that CFOs absolutely love, because you can take the AI budget and do something transformative that drops ROI to the bottom line immediately, almost you go from 60 to 99% accuracy, and suddenly there’s a lot of ROI on the line there. So I would look for places where, you know, people are clogging up the process. And then I was also encourage you to think about model operations and about having control over models as the future in an ensemble approach like that’s clearly where we’re going. It’s the lowest cost, best way to get the outcome you’re looking for. Rich. What do you got?
Rich Mautino 00:46:34
I really like talking about the solo chair process, because to me, that’s that’s something that when we talk to a lot of customers, they say expensive consultants come in and tell them, hey, you need to either simplify your process or find a way to integrate all these processes. And we know our customers well enough to know that simplifying those processes is rarely an option on the table. So how do you get it to all talk together? You look at any piece of technology and it gets more complex as time goes on, and that’s no different for our customers. So being able to make those processes seamless and kind of take the human away from that tennis ball bouncing back and forth that swivel chair, as it’s called, and basically weave it all together. You saw in the demo, there were probably 40 plus blocks as part of that, and none of those were filled or everything there is necessary to make the right decisions here. So reducing those inefficiencies of a human having to tie all of that together and kind of saving them for the key thing is the single, I think, biggest, wow, that’s so what that we get out of here, and that’s where we achieve a real competitive advantage in just extraction and run it head to head against, you know, other solutions that are out there. It’s a little bit more of a bake off. But when you bring it all together and make it less about, you know, 100 meter sprint and more about an Olympic, you know, competition, where you’ve got a multitude of events all happening kind of all at once, that’s really where the, you know, the compelling advantage exists.
Brian Weiss 00:47:59
Yeah, I’ll say a couple more things on that. Look, when you’re looking at solutions that process, you know, document one, ask, what happens when their solution gets it wrong, right? And because usually, when, especially newer companies, are probably wrapped around a third party API to a third party, you know, model that they don’t control, and they’re hoping, but you can’t, you can’t send those models some documents, and ask Amazon and make it better. It doesn’t right. So peel it back a little bit and understand what tools are being used. And ask yourself, what control do I have to make this better over time? Or am I just going to be renting something that I hope is good enough? And now I’m back into the whole BPO versus machine cycle, and I’ll say one last thing, and it’s, this is we are so excited about the about the possibility of agents, and AI driven, you know, human like, you know, folks who understand our business, right? This is a we’re all trying to get there. And hyper science is deeply involved in research in all of these departments. And the one thing that’s key is clean data, right? If you feed any of these agentic systems bad data, they will hallucinate faster, and you can misspell your own name. So you kind of got to focus on the garbage and garbage out in order to start those conversations we have. We see a lot of them that are way too advanced, and then you know why they stall, because they can’t get the data, they can’t get it clean, and they can’t guarantee that it’s clean. So look, we help. We help customers do that. And so if you’re on that journey, if you’re on that place where you’re looking to, you know, to deeply, mine your business data. Let us help. Let us help with that, that pipeline, I think Rich we’ve got, you know, look, if you’re ready, we’re here, we’re available. There’s a QR code. If that helps hyperscience.com we will send not only this, but also any other resources you’re looking for. And I think what I think GIA now we’re going to cut out to a Q and A right.
Gia Snape 00:49:53
That’s right. We received some questions from the audience. Thank you for engaging with us. We. Let’s start with the first one. How does hyper science handle complex documents, like multi page forms or handwriting?
Brian Weiss 00:50:07
Yeah, actually, that’s, that’s bread and butter stuff for for hyper science, like people often think like, oh, great, I’m gonna get a document and it’s gonna work fine. But no, actually, once you get a 50 page document with with a nested table that’s split across three different pages, that changes. That’s in a PDF that got sent to you in a stack with one file that’s actually got 15 pages, 15 different documents in it, and you have to figure out where one ends and the other begins, as well as split the other pages. And by the way, two of them are skewed badly because of like so that work I mentioned, we ship 35 plus models that are under the covers like hyperscience, does all of that work? Very, very good at that kind of, what we call computer vision management, of what’s coming through. And then you asked about handwriting. Handwriting is difficult. It’s hard. And you can imagine handwriting in multiple languages is hard. We have been doing deep learning, ml, AI, if you will, on our handwriting models for 10 plus years and continue to refine them for all of our customers. So there, we are very, very good at extracting handwriting correct whether it’s scribbles on a page or whether it’s inside a bounding box. It doesn’t really matter, like you know, but it is one of our specialties in the market. Yeah.
Gia Snape 00:51:23
Great and another question, does your solution easily integrate with existing claim systems?
Brian Weiss 00:51:29
Yes, great question. So we wouldn’t have the customers we have right now without being able to put inputs outputs, not just inputs and outputs to the systems, but also the data that’s required to make decisions, right? So whichever systems that lives into we’re, you know, we’ve been around for 10 years. We have some very large customers with complex systems. So when it comes to integrating, moving data in and out and through hyper science, or bringing data into hyper science from systems to make a decision, yeah, we have a robust set of capabilities there rich. I don’t think you’ve seen anything we haven’t been able to either have in the can or been able to very quickly, right? I mean that that that API world is, is priority robust,
Rich Mautino 00:52:11
Yeah, I would say that more of our customers have an integration than don’t. So it is very much table stakes. We can find a way
Gia Snape 00:52:23
Great and what level of accuracy is hyper science seeing with unstructured data extraction.
Brian Weiss 00:52:30
So when you say unstructured, if you mean like just fully scribbled handwriting and things like that, we get, we can get up to 99% right? And it’s because we’re constantly fine tuning that in from that that content, right? So again, when you say fully unstructured, it’s like there’s no anything. It’s going to be wildly unstructured. So we can drive up to 99% on that we’ve seen that. You know, the places where it gets interesting for for, for unstructured is actually see more of kind of slightly structured, long form documents. So where things are getting really interesting. For example, I mentioned, you know, the long form documents for claims in some financial industries. I mean, take, for example, a credit swap agreement. This is 5060, pages, and none of them are the same, and much of the data is is is delivered narratively, and we’ve helped a number of customers solve that where they were starting like, Hey, I’m just going to throw it at an LLM and tell it to go. I’m going to start writing 250 prompts to figure out what it wants. And then so what we do is we actually chunk that data up. And so we’ll section out which pieces are what, and we’ll basically pre annotate a long document, sometimes into a vector database, which gets even more interesting because you’re accumulating all that interesting data. But we see a lot of work in in, yes, fully unstructured, where you just ripping text off a page, right? And you’re trying to understand it, and you don’t know where it’s going to be. But this kind of long form, semi structured is, a pretty neat drill site for a lot of our customers. And yeah, the reason for that is because a lot of these use cases people thought were impossible like to just throw people at it like you’re not going to be able to automate it is, was the previous thinking. And so we, I don’t know if that answers the question, but I would not underestimate the accuracy rates for fully unstructured,
Rich Mautino 00:54:20
Yeah, and it all depends on the task too. Is, is accuracy a measurement of classifying what is the unstructured document? Is it looking for key medical terms in a doctor’s record, or classic extraction? Brian’s answer rings true for all of them, but we like to really understand what the outcome is and train the model in accordance with what you’re trying to achieve, rather than just kind of throwing it to a big bucket and hoping to get there, right?
Brian Weiss 00:54:47
Yeah, and that whole point of training them out, we came back to it right? Like, let’s get a model that does the job and needs to do really, really, really well, learns on the way and is inexpensive. Expensive to run. Like, I keep as a CTO. I’m like, Wow, that’s great, but you just burn my budget on a GPU solution that I didn’t need to spend money on. Like, why? Like, the efficiency of the task from a compute perspective kind of sometimes gets lost, and so the CTO has to sign a check in some of the excitement about AI. Let’s figure out the best tool for the job in the most efficient way possible.
Gia Snape 00:55:26
Okay, and we have a follow up question, actually. Can you share some details on how your platform ensures high accuracy for unstructured document types?
Brian Weiss 00:55:36
Well, I’ll go back to Rich’s answer too. When you say unstructured document types, and probably we should have, it’s like a back and forth conversation about what kinds of documents you’re looking at. So we do everything from a semi structured form, which would say, Okay, we kind of know where we kind of know what we’re looking for, but we don’t know where it lives. All right, that’s a I would, I would call that a semi structured but you’re kind of, you’re seeing the same structure. So power of attorneys, for example, okay, they’re always different, but, you know, the same information is generally contained in it, right? So it would be semi structured when you’re talking about fully unstructured documents, things that you know, handwriting all over a page, you know, full text extraction. We are training models specific to that task and and in the latest version of hyper science for fully unstructured text extraction, we are also doing what I will call accuracy tuning at the phrase level. So if you’re sort of think about text extraction, phrase extraction. We apply the same type of actually accuracy thresholds, but we’ll do it down to the to the actual text extraction itself. So that way you can put a human in the loop and gradually train around the specific model you’re looking for. Yeah. Now at the same time, I do want to, I do want to point out, like, a lot of like, a lot of lot of things, like, don’t forget, like, I like llms. I really do I like them until they’re wrong, right? So we’re using every piece of open source, and not open source technology out there, and we’re constantly benchmarking, not only against accuracy, fine tuning, but also cost it compared to, say, you know, a curated, small model approach, right? So it’s really an ensemble approach there.
Rich Mautino 00:57:25
Yeah, and we have the ability to leverage Qi QA tasks as well, basically take a random sampling of things that went all the way through and have a person validate it to make sure that our accuracy is what we think it is.
Brian Weiss 00:57:37
Yeah, that’s a good point rich, because we didn’t really talk about that model training and sort of model ops and part of it, but hyper spent, hyper spend a lot of money on an orchestration platform for what you would consider data science type work, right? So labeling, training, QA, that kind of work to get models, you know, up and running, and manage drift and that sort of thing. The beauty of that is, it’s not just like a bag of APIs that somebody hands you with some expensive consultants like, you know, non data science business users are the ones who are curating the accuracy of their models and managing it with Hyperscience.
Gia Snape 00:58:18
And very quickly, our last question for the day, could you provide insight into how your machine learning models continuously improve over time, particularly for handwriting recognition, for example, in cases where a date appears outside the bounding box, how does the system learn from a single correction to avoid repeating the same issue?
Brian Weiss 00:58:40
You want to take the bounding box one rich you want me to go at it? go at it?
Rich Mautino 00:58:42
Yeah, you can. We can probably tag team it. But bounding boxes aren’t an issue that we typically even see it fail on the first time, and that’s just by the way we train the models to begin with. But fine tuning is how we’re constantly kind of making small tweaks to the system to make sure that you know, if this person writes their Q, a little funny, for example, that it knows what that is and gets it right in the future. But Brian had some had some comment there.
Brian Weiss 00:59:06
One of the things we have built into the handwriting for extract, for extraction right, which is specific around handwriting models, is is built in fine tuning. And so what that does is it does the statistical analysis with some QA after the fact about when the machine thought it was wrong, but it was actually right. And so what we see is a lot of times, as you process data through, you end up calibrating when the machine thought it was right wrong, but was actually right, okay? And you sort of bring those down to a level. And so we’ll see, we’ll typically see a fine tuning accuracy bump in specific to handwriting extraction of 10 to 15% with a fine tuning process that really runs automatically as part of what’s built into the solution. You don’t have to go build that as a data scientist, right? And a lot of data scientists on the phone will know what I’m talking about. But you’re not actually retraining the model. You’re actually fine tuning the confidence levels. Within accurate, within weather was correct, but, but lack of confidence, and so you end up getting a pretty good kick in automation from our handwriting, and that’s why we start some of the, some of the ways we see most it’s not really accuracy, it’s more about automation. And so much like, yeah, we know our confidence in what’s right is higher. So I hope that answers the questions. By the way, we are more than available. Give us call. Be happy to talk through any your questions here and get more deep into architecture and fun things like that.
Gia Snape 01:00:31
Wonderful. And that brings us to the end of today’s session. A big thank you to our speakers for sharing their expertise and to all of you for joining us. Don’t forget to scan the QR code or click the link in the chat to learn more about Hyperscience and everything we discussed today, thanks again, and we hope to see you at our future events. Have a wonderful day.